TranSiGen: Novel Deep Generative Model for Phenotype-based Drug Screening
Phenotype-based screening is crucial for drug discovery, focusing on comprehensive cellular responses to drug candidates. This approach provides a more holistic understanding of disease mechanisms and potentially reveals novel drug targets and therapeutic avenues. High-throughput RNA sequencing technologies have facilitated the generation of large-scale perturbational gene expression profiles. Chemical-induced transcriptional profiles offer a comprehensive view of drug mechanisms, but inherent noise often obscures the true signals, hindering their potential for meaningful insights.
In a study published in Nature Communications on June 25, 2024, a research team led by ZHENG Mingyue from the Shanghai Institute of Materia Medica (SIMM) of the Chinese Academy of Sciences, proposed Transcriptional Signatures Generator (TranSiGen).
TranSiGen is a deep generative model which leverages self-supervised representation learning to denoise and reconstruct transcriptional profiles, enabling the inference of new perturbational profiles. The model offers a standardized way to characterize phenotypic information and demonstrates its efficacy in diverse downstream tasks.
In this study, extensive evaluations showed that TranSiGen outperforms existing models in inferring basal profiles, chemical-induced perturbational profiles, and corresponding differential expression genes (DEGs). As a type of unified representation, TranSiGen’s inferred DEGs effectively capture both cellular and compound features.
Furthermore, TranSiGen-derived representations demonstrated efficacy in diverse downstream tasks, including ligand-based virtual screening, drug response prediction, and phenotype-based drug repurposing. Notably, TranSiGen’s application in screening compounds against pancreatic cancer, with subsequent in vitro validation and high hit rates, demonstrated the power of its phenotype-based approach for identifying potent compounds.
This study highlighted the development of TranSiGen to mitigate the inherent noise in transcriptional profiles and provide a unified representation for phenotypic information, demonstrating its effectiveness in versatile downstream applications. TranSiGen’s phenotype-based approach for pancreatic cancer, with subsequent in vitro validation, showcases its promise for real-world drug discovery scenarios.
DOI: 10.1038/s41467-024-49620-3
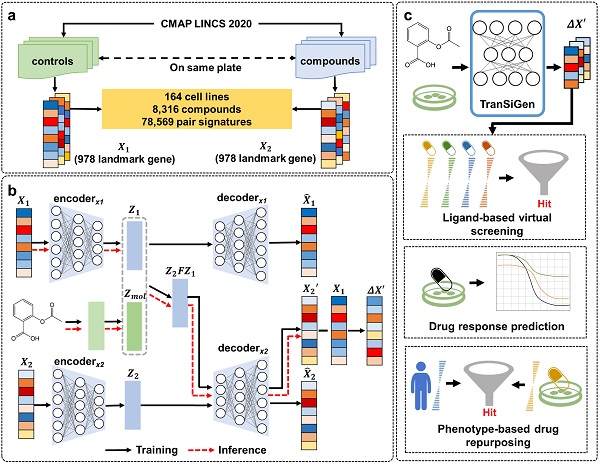
TranSiGen’s architecture and application. (Image by ZHENG Mingyue’s Laboratory)
Contact:
JIANG Qingling
Shanghai Institute of Materia Medica, Chinese Academy of Sciences
E-mail: qljiang@stimes.cn