Integrating Physical Knowledge and Data Augmentation for Protein–Ligand Interaction Scoring
Understanding protein-ligand interactions is crucial for drug discovery, yet developing robust methods for evaluating protein-ligand interactions has been a long-standing problem. While significant progress has been made, developing a scoring method with higher accuracy in practical application scenarios remains an open challenge.
In a study published in Nature Machine Intelligence on June 6, a research team led by ZHENG Mingyue from Shanghai Institute of Materia Medica (SIMM) of the Chinese Academy of Sciences, introduced a scoring approach called EquiScore. In virtual screening (VS) scenario and analogs ranking scenario, EquiScore demonstrated good predictive performance on unseen proteins. When used alongside different docking methods, EquiScore effectively enhanced their screening ability. Simultaneously, EquiScore is capable of capturing key inter-molecular interactions, providing useful clues for rational drug design.
In this study, researchers constructed a new dataset called PDBscreen using multiple data augmentation strategies, such as enlarging the positive sample size with near-native ligand binding poses and the negative sample size with generated highly deceptive decoys to avoid common biases. Then, leveraging the PDBscreen dataset, researchers trained a model using an equivariant heterogeneous graph architecture that incorporates different physical and prior knowledge about protein-ligand interaction.
In VS scenario, EquiScore outperformed 21 existing scoring methods on unseen proteins on two external datasets, DEKOIS2.0 and DUD-E. Remarkably, when considering only targets not seen during training, other deep learning-based models’ performance dropped significantly. In the analogs ranking scenario, EquiScore showed lower ranking ability than FEP+ among 8 different methods. Considering the significantly higher computational expenses required for FEP+ calculations, EquiScore demonstrated the advantage of more balanced speed and accuracy. Additionally, researchers found that EquiScore proves robust rescoring capabilities when applied to poses generated by different docking methods, and rescoring with EquiScore can enhance the VS performance of all evaluated methods.
In the ablation experiment section, researchers found that all modules in EquiScore significantly contribute to overall performance, and any removal would lead to performance degradation. However, roles of data augmentation and model design differ significantly across application scenarios. In VS, data augmentation methods notably enhance enrichment capability, with negative samples playing a major role. Interestingly, in the analogs ranking scenario, module contributions contrast with those in VS, while changes to the model architecture are more crucial than data augmentation. To further disentangle the contributions of the dataset and model architecture to performance, researchers also trained models with other architectures on PDBscreen. Even with the same training dataset, EquiScore still outperformed other compared models, underscoring the contribution of the model architecture.
Finally, by analyzing the model's interpretability, researchers found that EquiScore can capture key inter-molecular interactions, demonstrating its rationality and providing useful clues for rational drug design.
Robust prediction of protein-ligand interactions will provide valuable opportunities to understand the biology of proteins and determine their impact on future drug treatments. This study envision that EquiScore may contribute to a greater understanding of human health and disease, and discovery of novel medicines.
DOI: 10.1038/s42256-024-00849-z
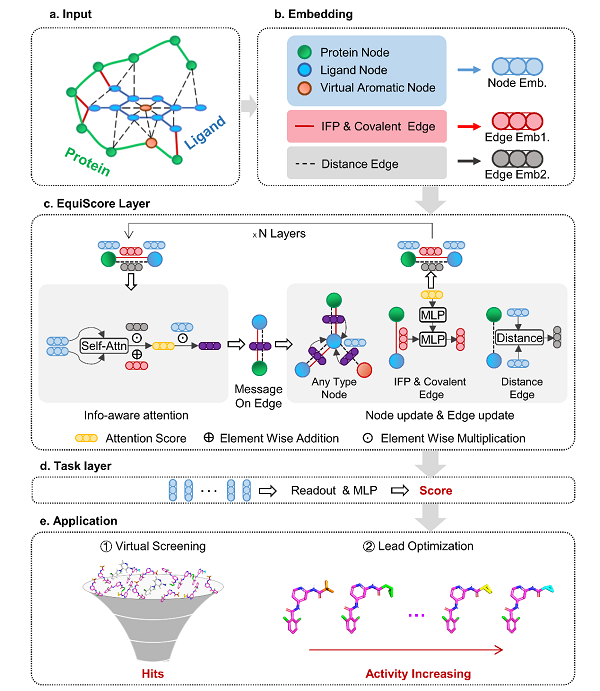
Overall architecture of EquiScore. (Image by ZHENG Mingyue 's Laboratory)
Contact:
JIANG Qingling
Shanghai Institute of Materia Medica, Chinese Academy of Sciences
E-mail: qljiang@stimes.cn