Advancing Chemical Text Mining: Fine-Tuning Large Language Models for Knowledge Extraction
Chemical literature contains a wealth of data, and extracting key chemical information through text mining techniques to build extensive databases can provide experimental chemists with detailed physical and chemical properties and synthesis routes. It can also offer computational chemists rich data and insights for model building and prediction. However, due to the complexity of chemical language and diversity of writing styles, extracting standardized and structured data from chemical literature is a highly challenging task.
In a study published in Chemical Science on June 7, 2024, a research team led by ZHENG Mingyue from Shanghai Institute of Materia Medica (SIMM) of the Chinese Academy of Sciences, conducted comprehensive study to explore the capabilities of various large language models (LLMs) across five key chemical text mining tasks. This work demonstrated the versatility and efficiency of fine-tuning LLMs for extracting chemical information from literature, providing valuable insights into practical applications of LLMs.
In this study, researchers evaluated various LLMs across five key tasks: compound entity recognition, reaction role labeling, metal-organic framework (MOF) synthesis information extraction, nuclear magnetic resonance (NMR) data extraction, and reaction synthesis paragraph-to-action sequence conversion. They explored multiple strategies using different LLMs, including guiding GPT-4 and GPT-3.5-turbo with zero-shot and few-shot prompt engineering, as well as parameter efficient fine-tuning or full parameter fine-tuning models like GPT-3.5-turbo, Llama3, Mistral, T5, and BART.
The research revealed that fully fine-tuned LLMs exhibited impressive performance, reducing reliance on prompting techniques meanwhile. The fine-tuned GPT-3.5-turbo excelled across all tasks, achieving exact match accuracies between 69% and 95%, outperforming specific models pre-trained on extensive domain-specific data. Additionally, open-source models like Mistral and Llama3, once fine-tuned, demonstrated competitive performance, showcasing the potential of LLMs in mining private data locally.
This study underscored the flexibility, robustness, efficiency, and low-code nature of fine-tuned LLMs in chemical text mining. In light of these properties, fine-tuning LLMs can emerge as a powerful method for information extraction, accelerating data collection and scientific discovery across various fields.
DOI: 10.1039/D4SC00924J
Links: https://doi.org/10.1039/D4SC00924J
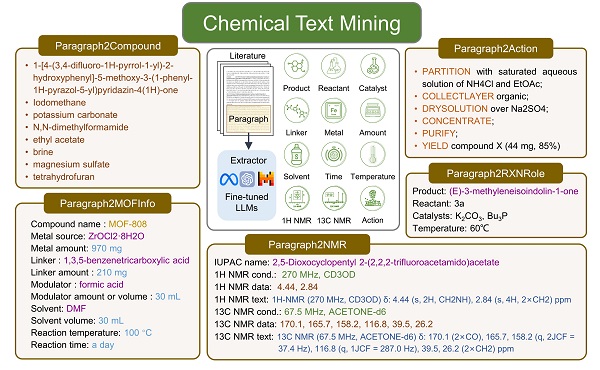
Schematics of cheminformatics insights extracted from paragraphs with LLMs. (Image by ZHENG Mingyue 's Laboratory)
Contact:
JIANG Qingling
Shanghai Institute of Materia Medica, Chinese Academy of Sciences
E-mail: qljiang@stimes.cn